Understanding the Power Law Distribution
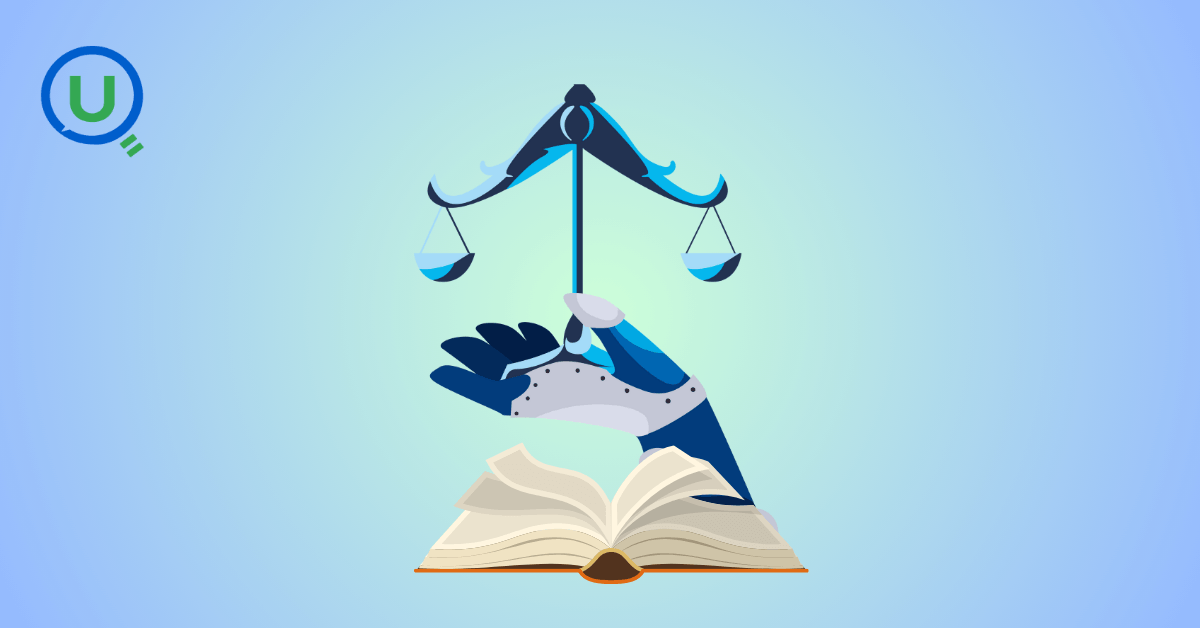
Ready to transform your data strategy with cutting-edge solutions?
Check out the below post I found on LinkedIn :
What caught my attention is the statement highlighted in Yellow. Following questions hit me almost instantly :
1. What events in the real world follow normal distribution?
2. If Content hits (read virality) doesn't follow normal distribution then which "one" does it follow?
3. What other kinds of statistical distributions exist and their real world use cases?
Let's try to answer the above questions in this blog.
Why Content Success Doesn't Follow a Normal Distribution?
Normal Distribution Basics: In a normal distribution, data points cluster around a mean (average) value with symmetrical tails on both sides. This kind of distribution is common in traits like human height or IQ, where most values are close to the mean and extreme values are rare.
Content Engagement Patterns: In content marketing, engagement patterns are different. Rather than clustering around an "average level of success," we often see that a small percentage of content pieces drive the majority of engagement and traffic. This pattern aligns with the Pareto Principle, or the 80/20 rule, where roughly 20% of content generates 80% of results.
Power-Law Distribution: Instead of a normal curve, content hits tend to follow a power-law distribution, which has a "long tail." This means that while a few pieces of content (like viral posts or hit videos) perform exceptionally well, the majority of content sees only modest engagement. In the LinkedIn post's case, a single video attracted 40% of total subscribers—a huge outlier compared to typical performance.
Examples of Outliers in Content and Media :
Social Media Posts: On platforms like Twitter or Instagram, a small number of posts might go viral, reaching millions, while the vast majority receive minimal engagement. For instance, a single tweet might gather hundreds of thousands of likes and retweets, while most tweets by the same user barely reach a fraction of that.
Blog Traffic: In a blog with hundreds of posts, it’s often observed that only a handful of “pillar” articles drive the bulk of traffic. These outlier articles often rank well on search engines or resonate uniquely with readers, creating a disproportionately large impact compared to the majority of posts.
E-commerce Products: A small percentage of products in an e-commerce store may generate most of the sales and revenue, while many products see little to no purchases. This is why companies focus on identifying and promoting bestsellers.
Fundamentals of Power Law Distribution :
In practical terms, power-law distributions describe situations where:
A few items have very high values (e.g., a few videos go viral).
Many items have low or moderate values (e.g., most videos get average or low views).
Real world phenomenon following the Power Law Distribution :
City Sizes: In most countries, a few cities (like New York or Mumbai) have very large populations, while the majority of cities are much smaller.
Wealth Distribution: A small percentage of people hold a large portion of global wealth, while most people have moderate or low wealth.
Website Traffic: A handful of websites (like Google and YouTube) get most of the traffic, while millions of sites receive only a fraction.
Word Frequencies: In any language, a few words are used very frequently, while many words are rarely used.
Common distributions in Nature :
Here's a visual comparison of the different distributions:
Power-Law Distribution (Top Left): Notice how it has a long tail on the right, meaning that high values are possible but rare. This type of distribution is common in phenomena with outliers, like content virality, where a few pieces achieve massive reach, while most remain low.
Poisson Distribution (Top Right): It clusters around a central value (in this case, around 10 events) and quickly drops off. It's suited for events that occur randomly over a fixed interval, like the number of customers arriving in a store per hour.
Binomial Distribution (Bottom Left): The probability of different numbers of successes in a series of trials (here, with n=50 and p=0.2) forms a distribution similar to Poisson but is bounded by the total number of trials, unlike the unbounded nature of Power-Law.
Normal Distribution (Bottom Right): Symmetrical and bell-shaped, with most values clustering around the mean. This distribution is common in traits like human height, where extremes are rare and values are concentrated around an average.
The Power-Law Distribution stands out due to its asymmetry and long tail, making it ideal for modeling situations with a few dominant outliers, like viral content or wealth distribution. This highlights why certain events are not well-explained by traditional distributions like Poisson or Normal.
Ready to Experience the Future of Data?
You Might Also Like
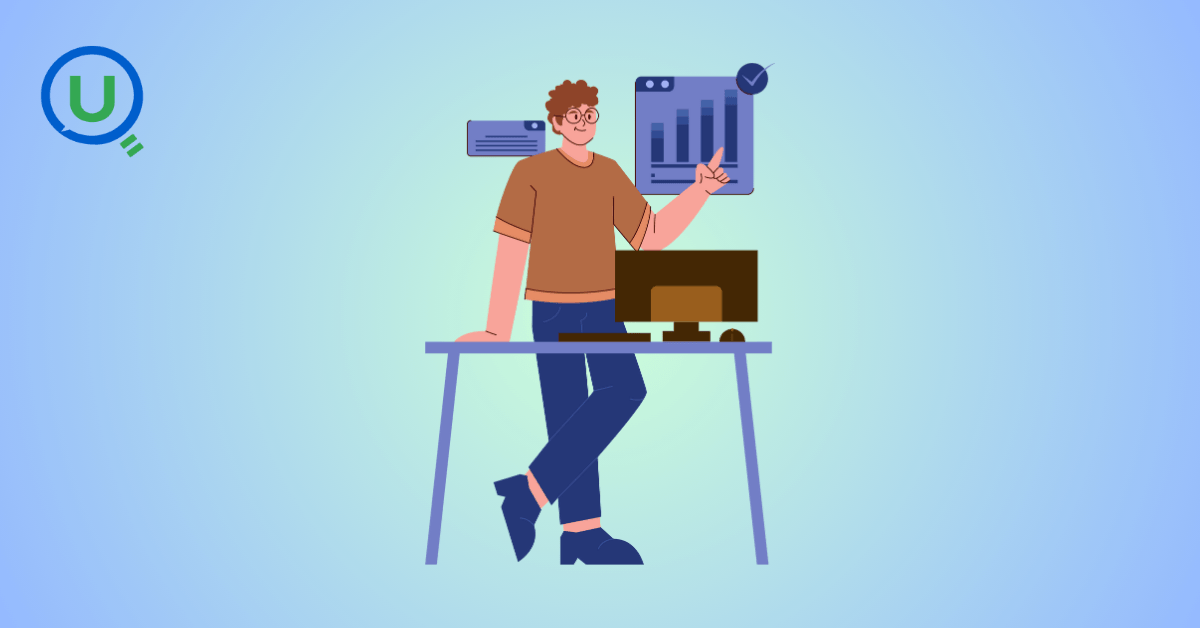
This is the first in a five-part series detailing my experience implementing advanced data engineering solutions with Databricks on Google Cloud Platform. The series covers schema evolution, incremental loading, and orchestration of a robust ELT pipeline.
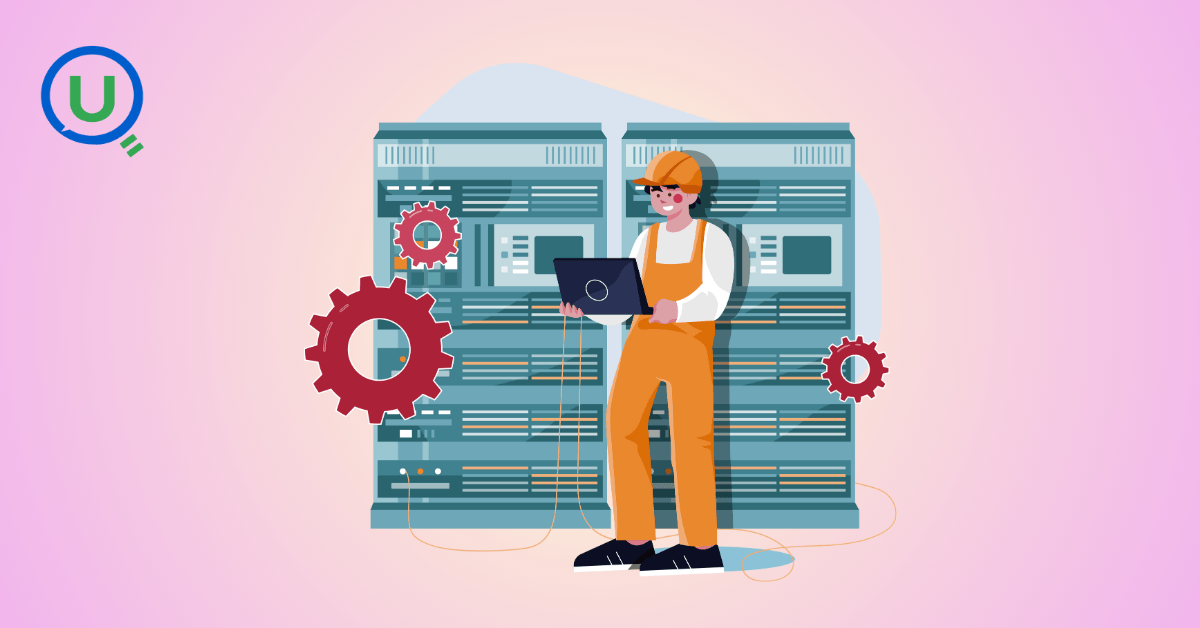
Discover the 7 major stages of the data engineering lifecycle, from data collection to storage and analysis. Learn the key processes, tools, and best practices that ensure a seamless and efficient data flow, supporting scalable and reliable data systems.
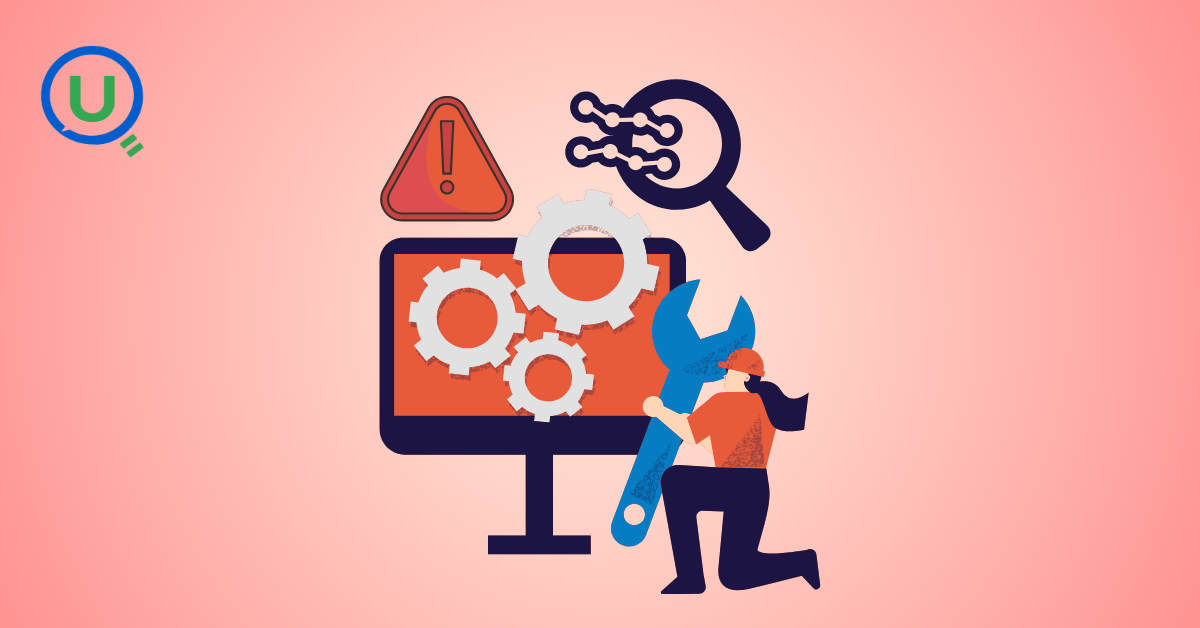
This blog is troubleshooting adventure which navigates networking quirks, uncovers why cluster couldn’t reach PyPI, and find the real fix—without starting from scratch.
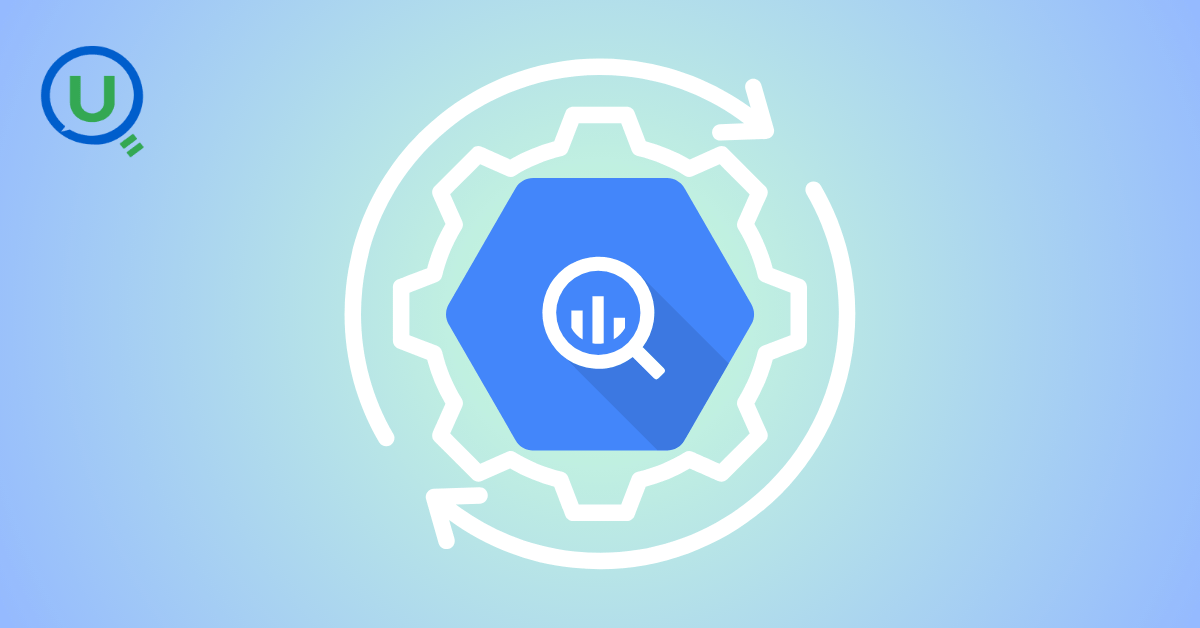
Explore query scanning can be optimized from 9.78 MB down to just 3.95 MB using table partitioning. And how to use partitioning, how to decide the right strategy, and the impact it can have on performance and costs.
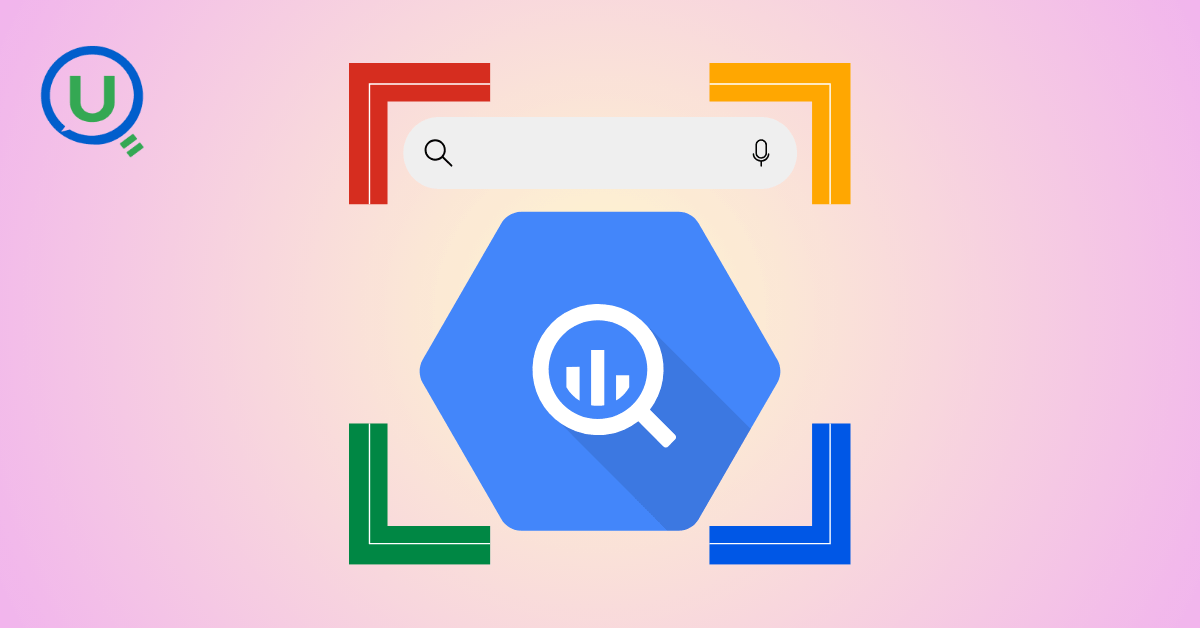
Dive deeper into query design, optimization techniques, and practical takeaways for BigQuery users.
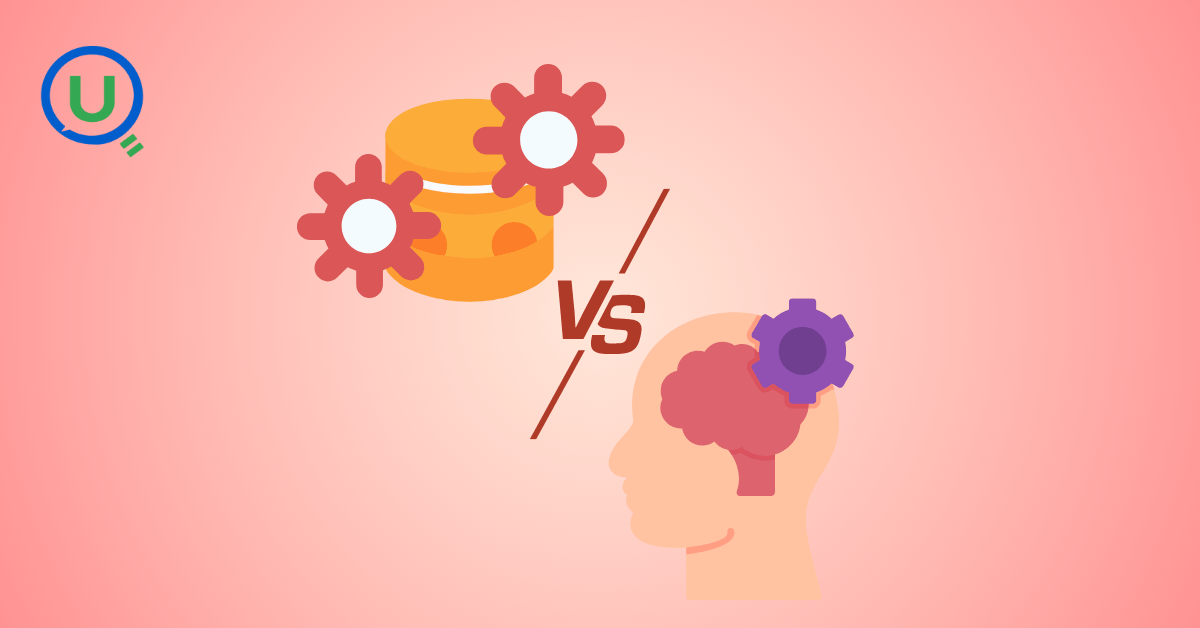
Wondering when to use a stored procedure vs. a function in SQL? This blog simplifies the differences and helps you choose the right tool for efficient database management and optimized queries.
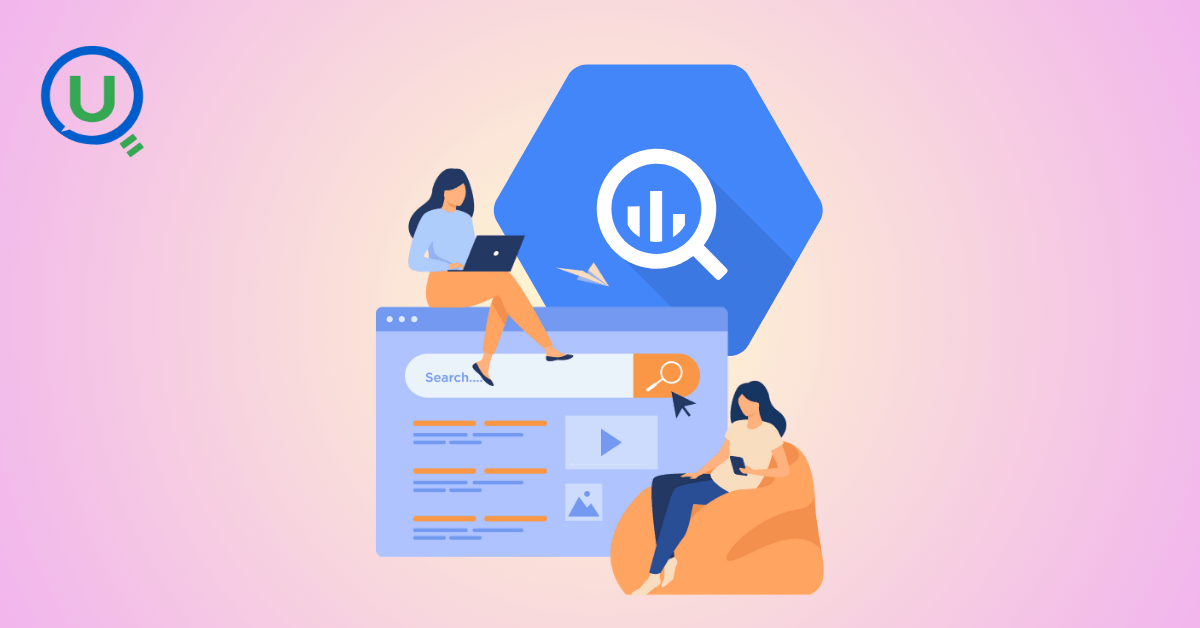
Discover how BigQuery Omni and BigLake break down data silos, enabling seamless multi-cloud analytics and cost-efficient insights without data movement.
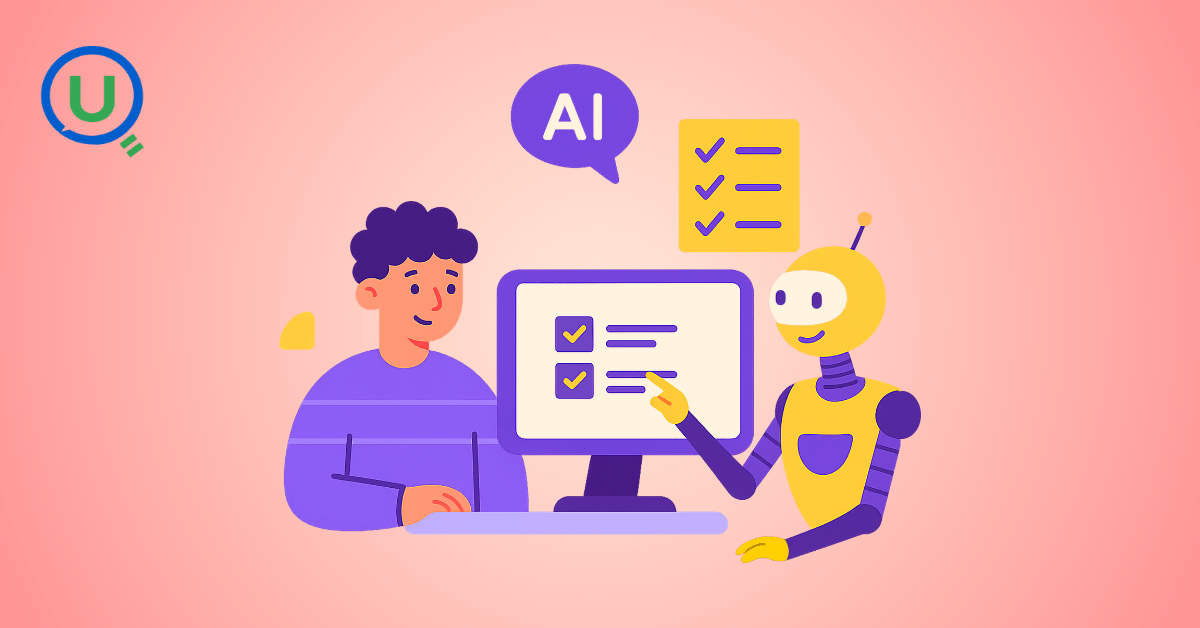
In this article we'll build a motivation towards learning computer vision by solving a real world problem by hand along with assistance with chatGPT
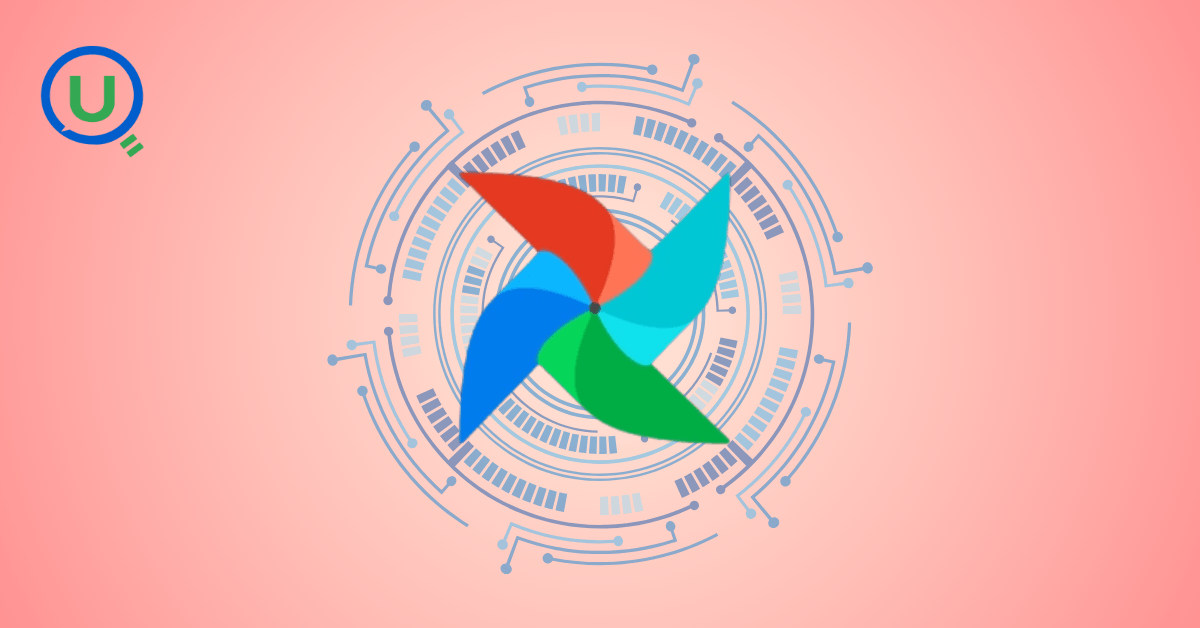
This blog explains how Apache Airflow orchestrates tasks like a conductor leading an orchestra, ensuring smooth and efficient workflow management. Using a fun Romeo and Juliet analogy, it shows how Airflow handles timing, dependencies, and errors.
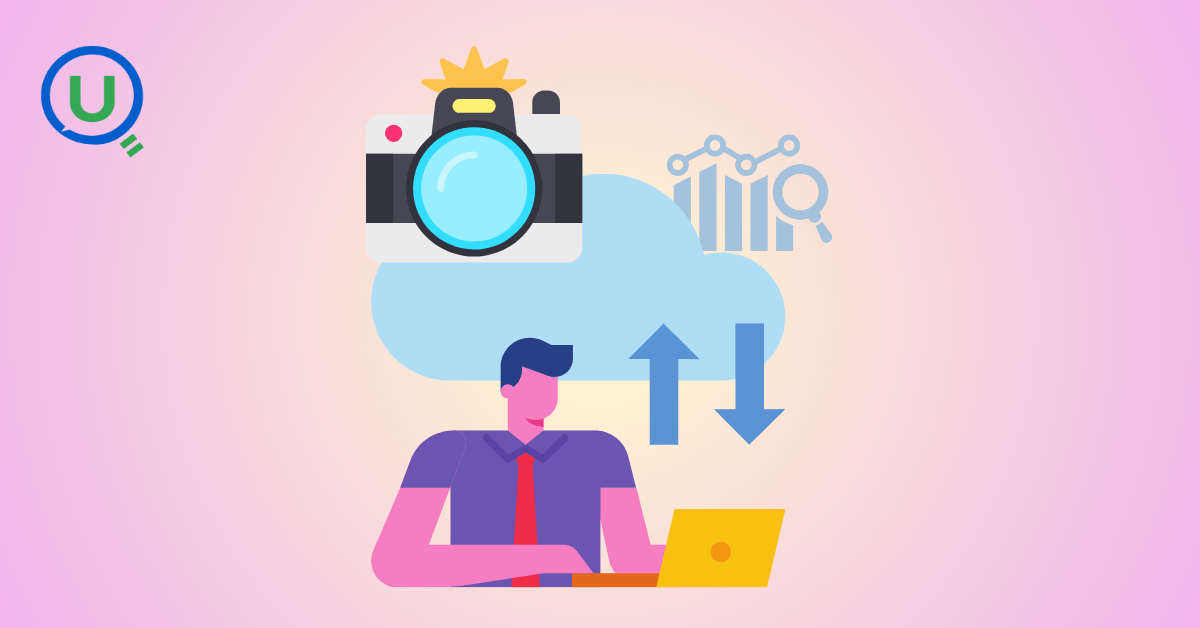
The blog underscores how snapshots and Point-in-Time Restore (PITR) are essential for data protection, offering a universal, cost-effective solution with applications in disaster recovery, testing, and compliance.
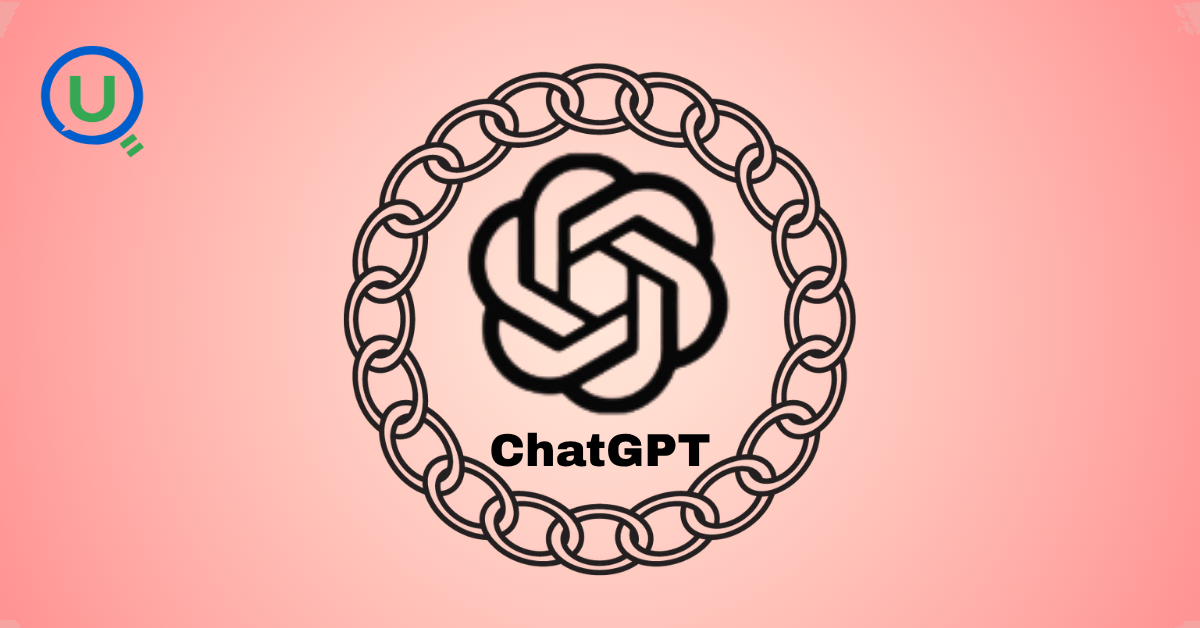
The blog contains the journey of ChatGPT, and what are the limitations of ChatGPT, due to which Langchain came into the picture to overcome the limitations and help us to create applications that can solve our real-time queries
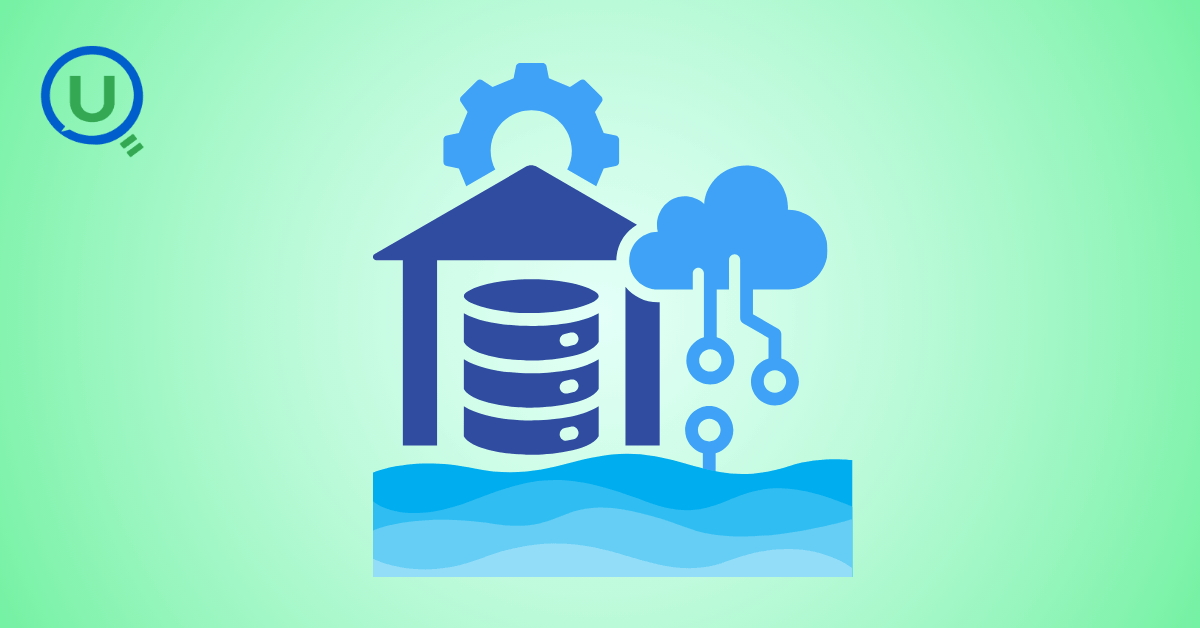
This blog simplifies the complex world of data management by exploring two pivotal concepts: Data Lakes and Data Warehouses.
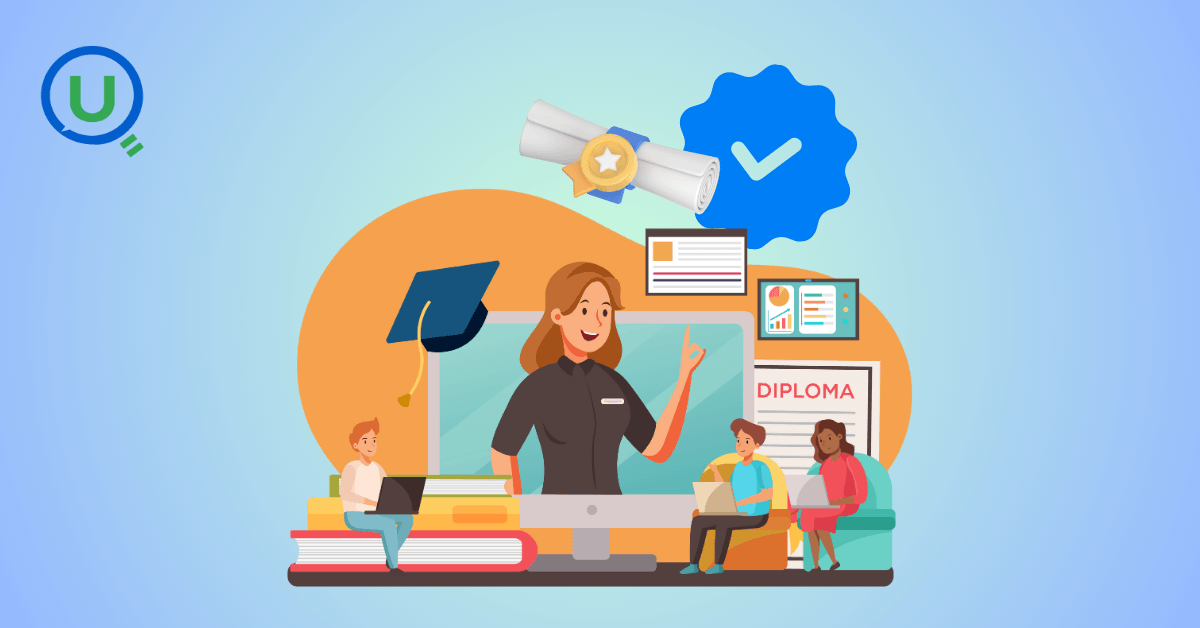
An account of experience gained by Enqurious team as a result of guiding our key clients in achieving a 100% success rate at certifications
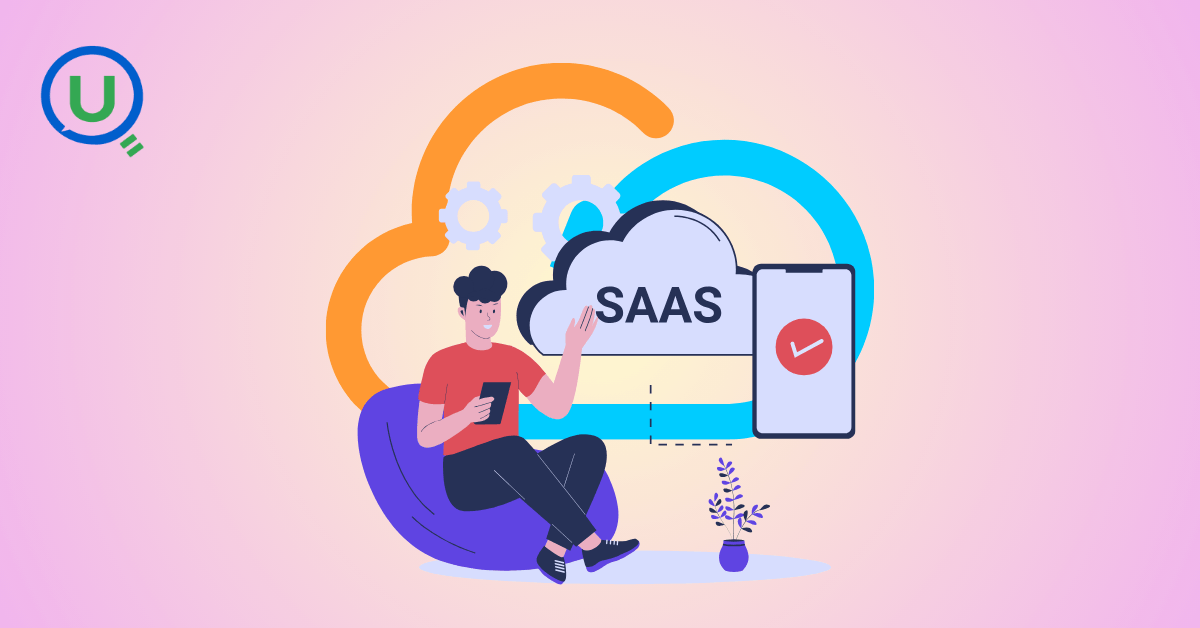
demystifying the concepts of IaaS, PaaS, and SaaS with Microsoft Azure examples
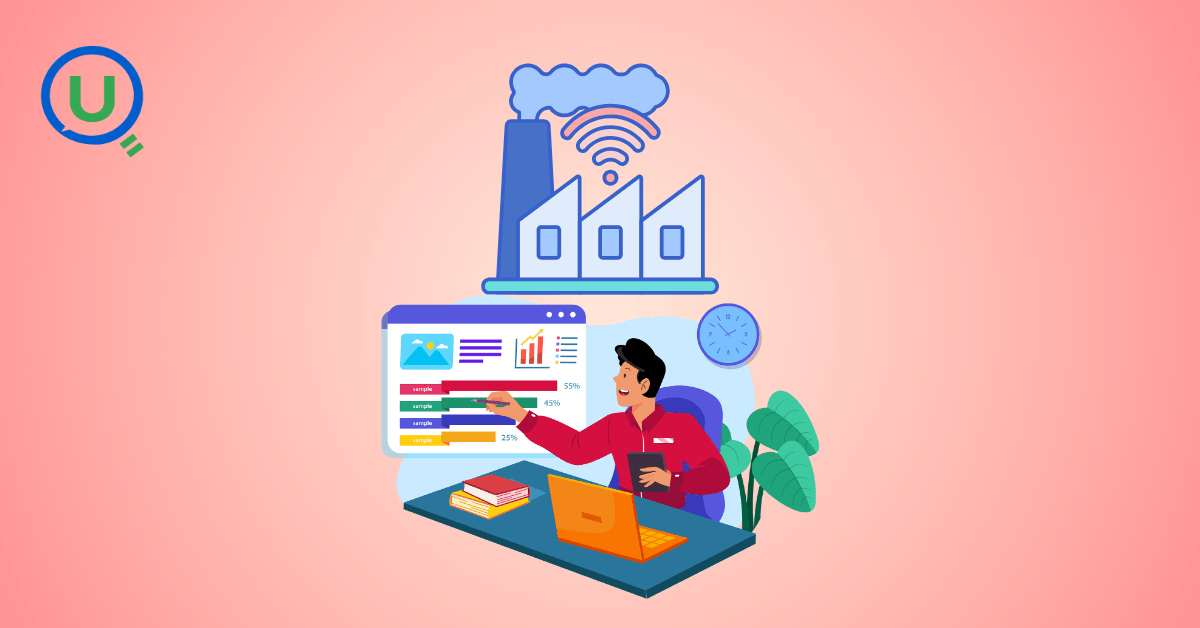
Discover how Azure Data Factory serves as the ultimate tool for data professionals, simplifying and automating data processes
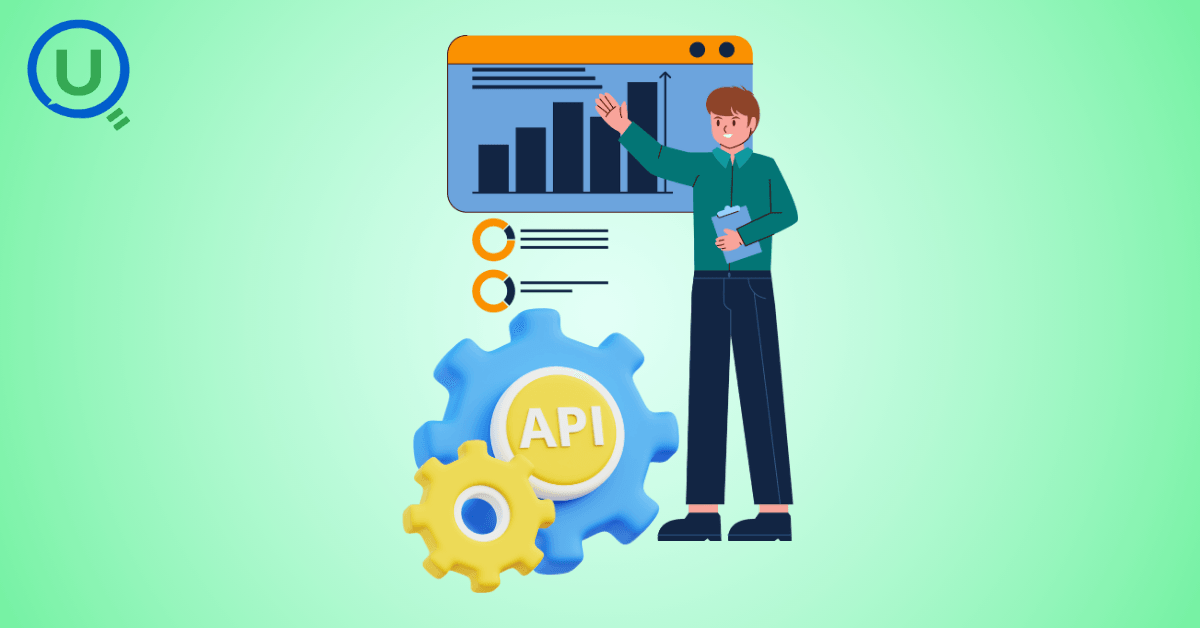
Revolutionizing e-commerce with Azure Cosmos DB, enhancing data management, personalizing recommendations, real-time responsiveness, and gaining valuable insights.
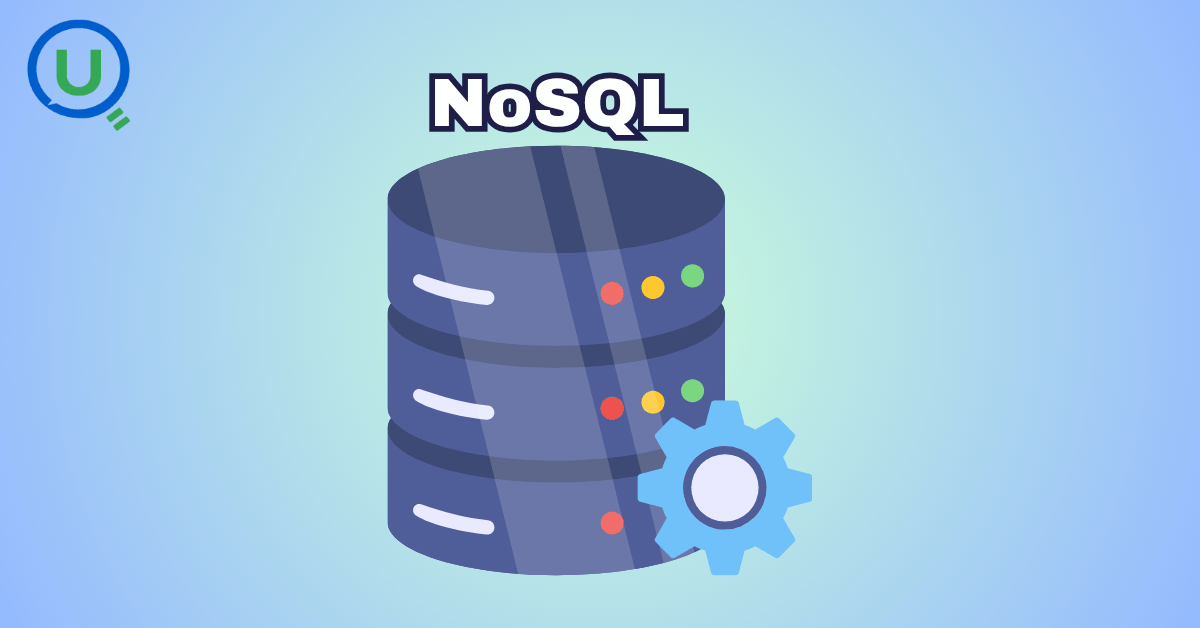
Highlights the benefits and applications of various NoSQL database types, illustrating how they have revolutionized data management for modern businesses.
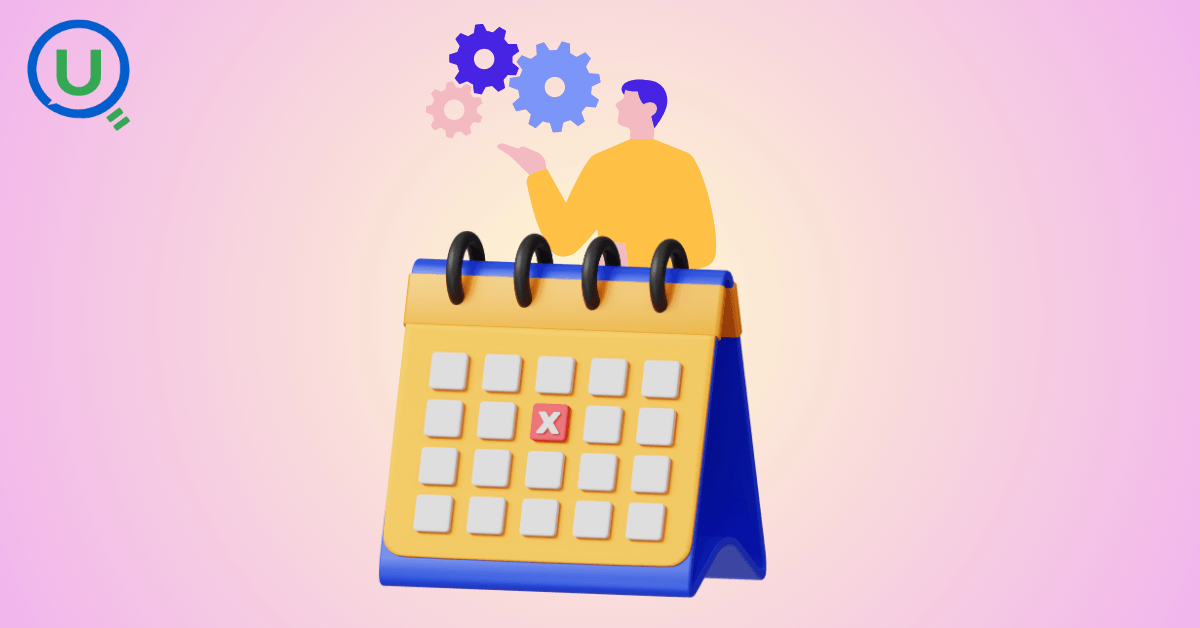
This blog delves into the capabilities of Calendar Events Automation using App Script.
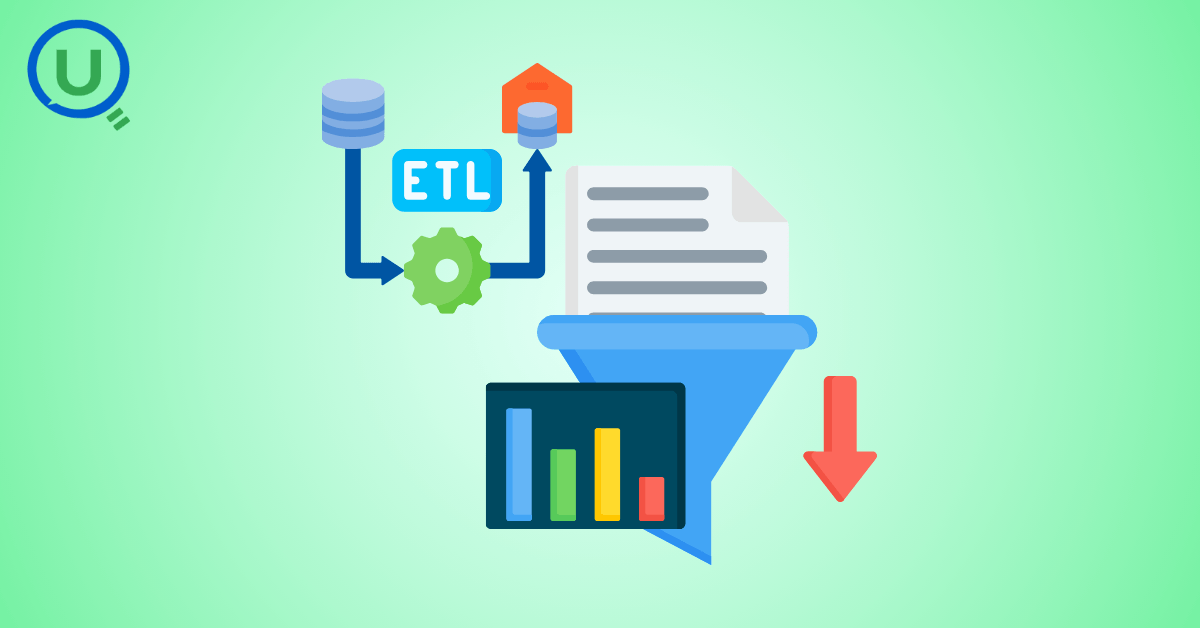
Dive into the fundamental concepts and phases of ETL, learning how to extract valuable data, transform it into actionable insights, and load it seamlessly into your systems.
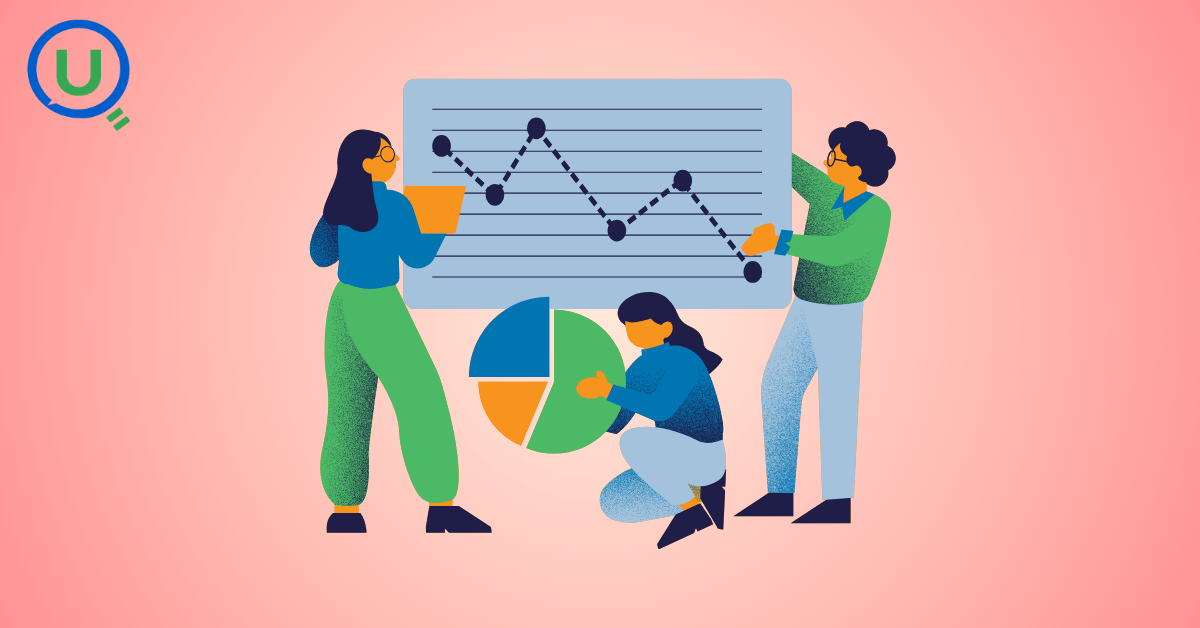
An easy to follow guide prepared based on our experience with upskilling thousands of learners in Data Literacy
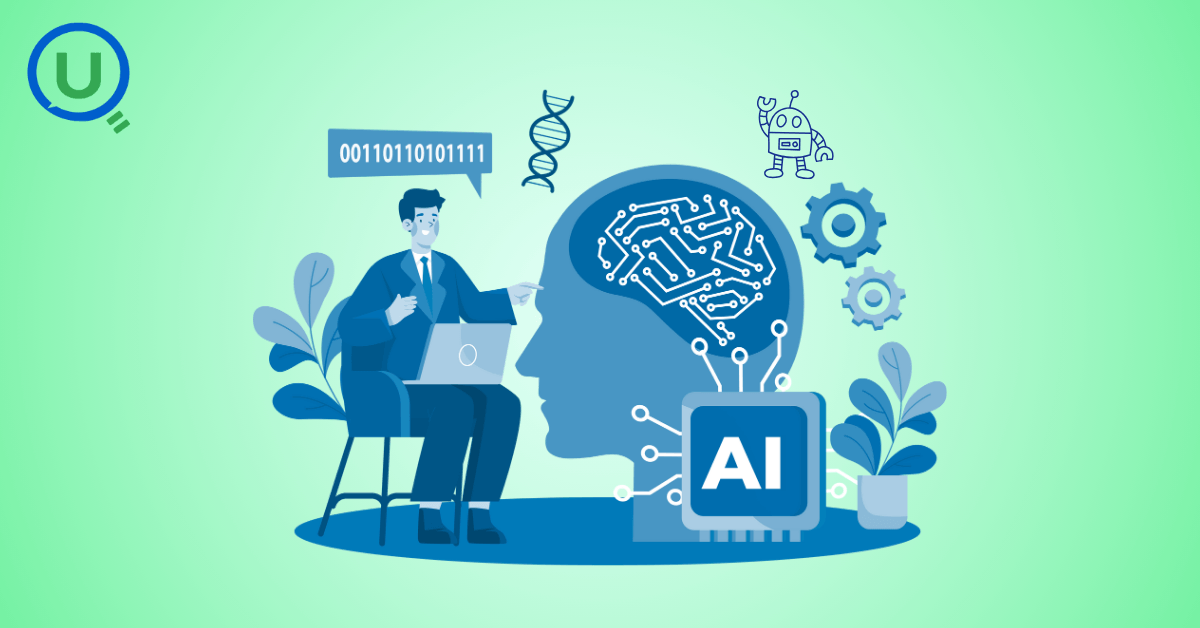
Teaching a Robot to Recognize Pastries with Neural Networks and artificial intelligence (AI)
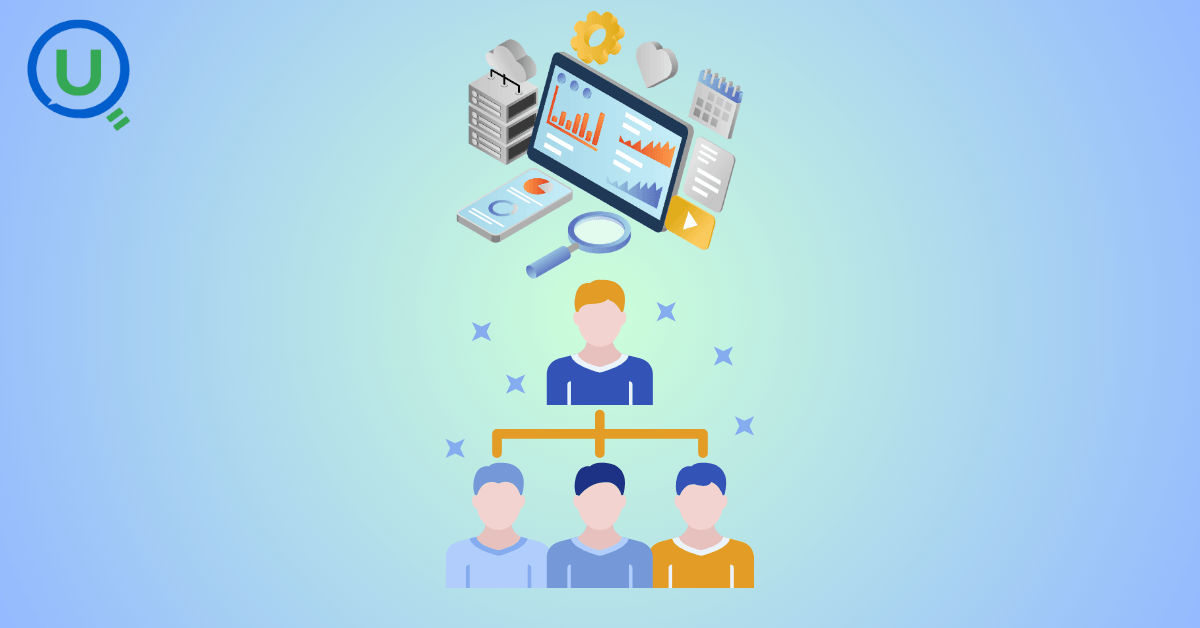
Streamlining Storage Management for E-commerce Business by exploring Flat vs. Hierarchical Systems
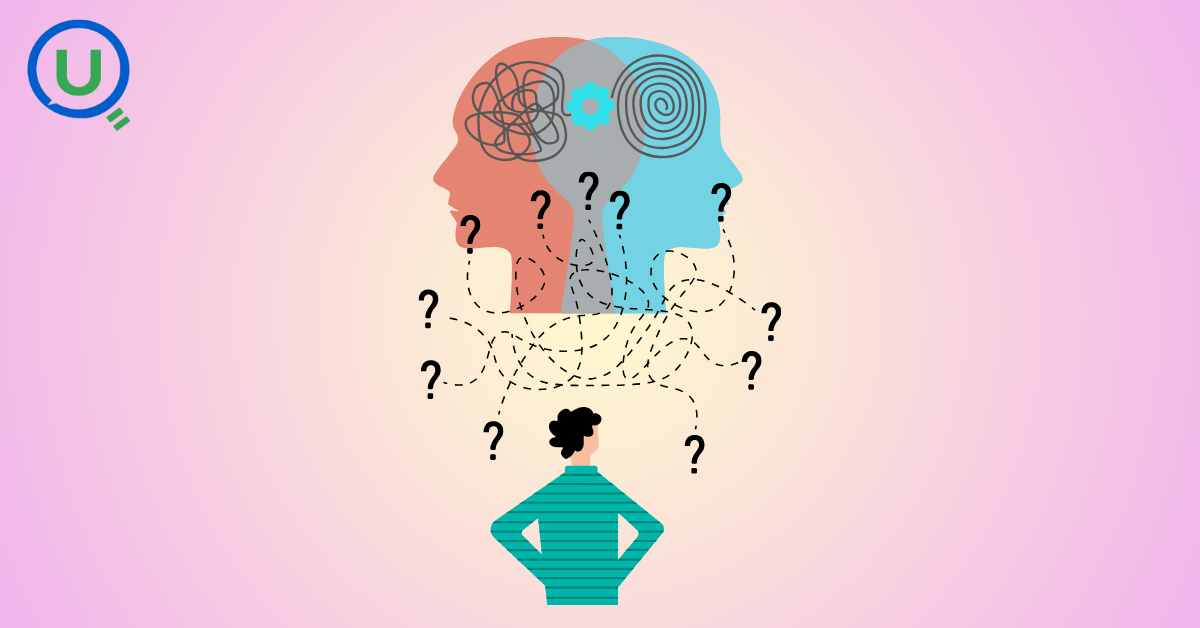
Figuring out how Cloud help reduce the Total Cost of Ownership of the IT infrastructure
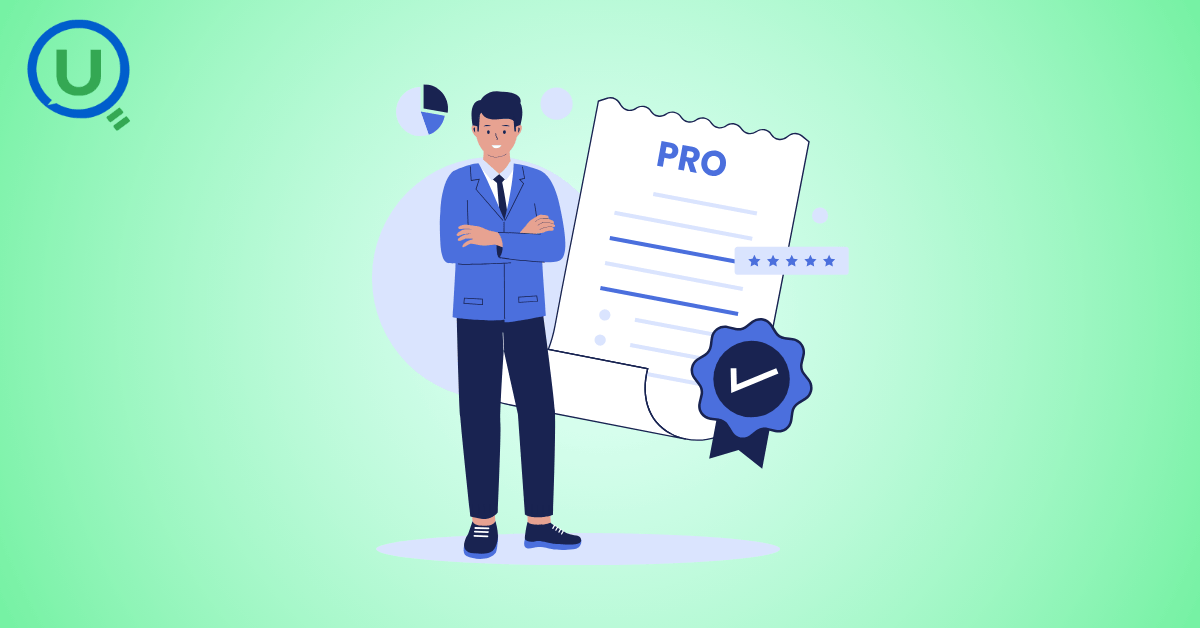
Understand the circumstances which force organizations to start thinking about migration their business to cloud