10 Key Data Science Trends To Watch Our For in 2025
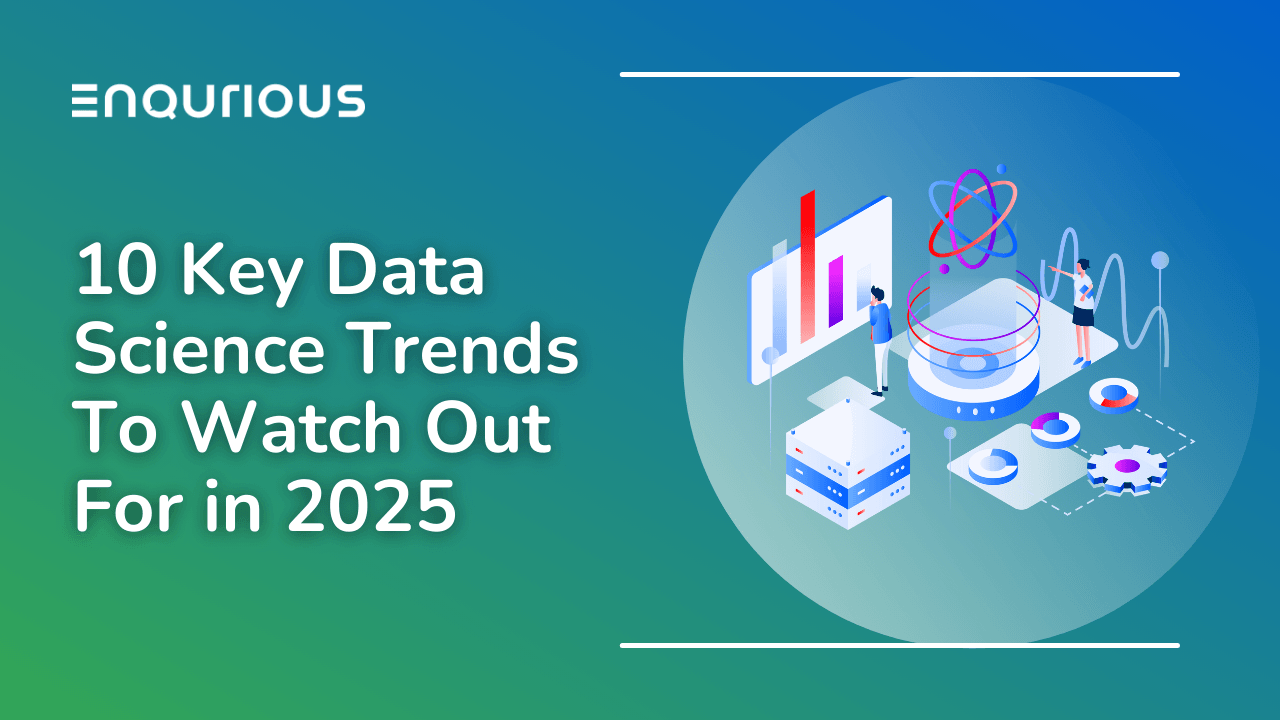
Data science continues to evolve at an unprecedented pace, with new techniques, tools, and technologies emerging regularly. As we move into 2025, businesses and data professionals must stay on top of the latest trends to remain competitive and drive innovation. Data science is no longer just about analyzing historical data; it's about leveraging advanced techniques to forecast future trends, automate decisions, and provide actionable insights.
In this blog, we’ll explore what is the trend of data science in 2025 and what these trends mean for the industry.
1. Increased Adoption of Generative AI
Generative AI is revolutionizing various fields, and data science is no exception. With the rise of models like GPT-4, GANs (Generative Adversarial Networks), and other generative models, data scientists are now able to create data, text, images, and even video content that mirrors real-world data. In 2025, expect more businesses to leverage generative AI for content creation, data augmentation, and simulation.
Why it’s important: Generative AI will help businesses optimize data-driven decision-making by creating synthetic data for training models, reducing data privacy concerns, and making predictions with more precision.
2. Automated Machine Learning (AutoML)
Machine learning is the backbone of data science, but the process of building, training, and fine-tuning models can be time-consuming and requires deep expertise. AutoML platforms have emerged to automate many aspects of this process. By simplifying the workflow, AutoML empowers data scientists to create machine learning models faster and with less manual intervention.
Why it’s important: With AutoML, organizations can build and deploy models more quickly, ensuring that they stay ahead of the competition while allowing data scientists to focus on higher-level tasks.
3. Advancements in Natural Language Processing (NLP)
Natural Language Processing (NLP) has witnessed incredible advancements in recent years, with deep learning models enabling machines to understand, generate, and interpret human language. By 2025, NLP will continue to transform industries, particularly customer service, content creation, and data extraction from unstructured sources. With improved sentiment analysis, real-time translation, and enhanced chatbots, NLP's capabilities will only expand.
Why it’s important: NLP will make human-computer interaction smoother and more intuitive, enabling businesses to derive valuable insights from unstructured data like social media, reviews, and customer feedback.
4. AI-Driven Analytics
Artificial Intelligence (AI) is increasingly being integrated into analytics platforms, enabling organizations to gain deeper insights, faster. AI-powered analytics tools can automatically detect patterns in data, predict future trends, and even recommend actions based on analysis. In 2025, we’ll see more companies embracing AI-driven analytics to streamline decision-making processes across all departments.
Why it’s important: AI-powered analytics reduces human error, uncovers hidden patterns, and provides actionable insights that can drive innovation and efficiency in business operations.
5. Ethics and Explainability in AI
As AI models become more sophisticated, the need for transparency and ethical considerations has grown. By 2025, businesses and regulatory bodies will place a greater emphasis on the explainability of AI models. Data scientists will need to prioritize building models that are not only accurate but also interpretable, ensuring that decisions made by AI are ethical and can be understood by humans.
Why it’s important: AI explainability builds trust and ensures that AI is used in a responsible, transparent manner, particularly in industries such as healthcare, finance, and legal systems.
6. Edge Computing and Data Science
Edge computing refers to the practice of processing data closer to the source rather than sending it to centralized servers. In data science, this trend will become more significant in 2025, especially with the proliferation of IoT devices. Edge computing allows data scientists to perform real-time analytics on data generated by sensors and devices, enabling faster decision-making and reducing latency.
Why it’s important: Edge computing enables real-time insights, which is particularly important in industries like manufacturing, healthcare, and autonomous vehicles, where quick data processing is critical.
7. Responsible Data Science and Privacy Concerns
With growing concerns over data privacy and security, responsible data science will become an essential part of every data-driven project. Data scientists will need to adhere to stricter regulations and follow ethical guidelines while working with sensitive information. By 2025, expect a more significant push towards adopting privacy-preserving methods such as differential privacy and federated learning.
Why it’s important: Ensuring data privacy and security is paramount in maintaining user trust, particularly as data collection becomes more pervasive in modern business models.
8. Cloud-Based Data Science Platforms
The cloud continues to be a key enabler of data science. By 2025, cloud-based platforms like AWS, Google Cloud, and Microsoft Azure will see even wider adoption, as they offer scalability, flexibility, and cost-effective resources for data scientists. These platforms allow teams to collaborate more effectively, access vast amounts of data, and use powerful computing resources for training complex models.
Why it’s important: Cloud-based platforms provide the infrastructure needed to handle the growing demands of data science while allowing businesses to scale their operations efficiently.
9. Integration of AI with Business Intelligence (BI)
By 2025, business intelligence (BI) tools will increasingly incorporate AI and machine learning capabilities. This integration will allow companies to gain insights from their data faster, by automating data analysis and generating predictive insights in real time. AI-powered BI tools will help data scientists and business analysts work in tandem to optimize business decisions.
Why it’s important: AI integration with BI will drive smarter business decisions, allowing companies to optimize marketing campaigns, manage supply chains, and improve customer experiences with actionable insights.
10. Data Governance and Data Management
As businesses handle larger volumes of data, data governance will play an even more crucial role in ensuring the quality, security, and compliance of data. Data scientists will be expected to work closely with data engineers and governance teams to ensure that data used for training machine learning models meets all required standards.
Why it’s important: Effective data governance ensures that data used for machine learning models is accurate, reliable, and compliant with regulations, making it essential for building trustworthy AI systems.
Conclusion
The trend of data science in 2025 is marked by tremendous technological advancements that are transforming how organizations analyze and use data. From AI-driven analytics and generative AI to the increased focus on ethics and privacy, data science will continue to push the boundaries of what’s possible. As these trends take shape, professionals and businesses alike will need to stay ahead of the curve to leverage these innovations for competitive advantage.
At Enqurious, we understand the importance of staying up-to-date with these emerging trends. Our hands-on, scenario-driven learning experiences equip data professionals with the skills needed to succeed in a rapidly evolving data landscape. Whether you’re looking to upskill or transition into data science, Enqurious offers a variety of training programs that focus on real-world applications, helping you build expertise that will drive your career forward in 2025 and beyond.