A Complete Step-by-Step Guide to Data Analytics Framework
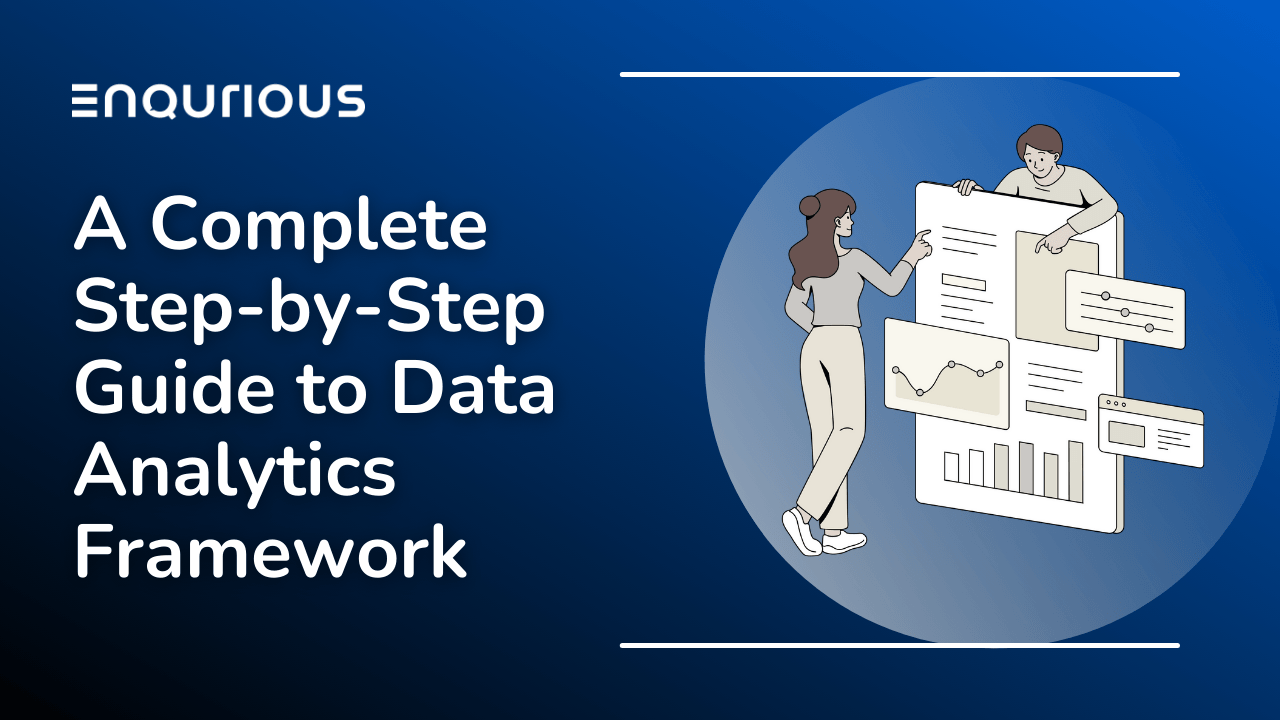
In today's data-driven world, knowing how to make sense of information is a crucial skill. We’re surrounded by test scores, app usage stats, survey responses, and sales figures — and all this raw data on its own isn’t helpful unless we know what to do with it. That’s where a data analytics framework comes in. It brings structure to the chaos and guides us in transforming messy data into meaningful insights.
Think of the data analytics framework as your personal GPS in the world of data. It tells you where to start, what turns to take, and how to reach your destination: better decisions, backed by facts.
If you’re a beginner, don’t worry. This guide is designed to keep things clear, simple, and a bit fun. Whether you're analyzing exam scores, improving customer service, or simply trying to understand why you binge-eat cookies during deadlines — the process is the same.
We’ll walk through each step using a super-relatable example: a student trying to figure out why their math score took a nosedive. No spreadsheets required (unless you want to). By the end, you’ll understand what to do with your data, how to do it, and why each step actually matters.
Step 1: Define the Problem
To begin any analysis using a data analytics framework, it’s essential to clarify what you’re trying to solve. A well-defined problem sets the direction of the entire analysis and ensures that the process remains focused and purposeful.
Every impactful data project begins with a well-defined problem. You can’t solve what you don’t understand. Instead of vague goals like “improve performance,” you need specific, focused questions that guide your analysis.
Start by asking: What is the business trying to achieve? Who are the stakeholders? What decisions will be influenced by the analysis.
Example:
Let’s simplify it. Imagine a student who scores poorly in their math exam. Instead of saying, “I need to get better at math,” they define their problem more clearly: “I need to improve my algebra score, where I lost 15 out of 30 marks.”
The better the problem is framed, the easier it becomes to solve.
Step 2: Gather Relevant Data
The second step in any data analytics framework involves collecting all relevant data that can help answer the problem. This includes both quantitative and qualitative data.
Once your problem is clear, it’s time to collect the data that can help you answer it. This might come from multiple sources — past exam results, study logs, attendance records, even stress levels.
Don’t restrict yourself to just numbers. Qualitative inputs like teacher feedback or self-reflection can add valuable context.
Example:
The student gathers:
- Previous exam papers
- Homework grades
- Time spent on different topics
- Hours of sleep before each test
- Notes from math class and tutoring sessions
Together, these give a full picture of what’s going on.
Step 3: Clean and Prepare the Data
In any data analytics framework, raw data must be cleaned and prepared before it can be analyzed effectively. This step ensures that your insights are based on reliable and accurate data.
Now that we’ve got data, let’s face it — it’s going to be messy. This step is about tidying up so the analysis is trustworthy.
You’ll handle missing values, inconsistent formats, typos, or duplicate entries. Think of this as prepping ingredients before cooking. Even the best recipe can fail with bad ingredients.
Example:
The student notices that some scores are listed as “15/20” and others as “75%.” They convert everything into percentages for consistency. A few duplicate entries? Deleted. Now the dataset is clean, consistent, and ready for analysis.
Step 4: Explore and Analyze the Data
With a clean dataset, the data analytics framework now moves to exploring the data for patterns, trends, and correlations that provide insights into the problem.
This is the fun part — digging into the data to see what it reveals. It's where confusion starts turning into clarity.
Use simple stats, visualizations, and maybe a pivot table to look for trends and patterns. Which topics are pulling scores down? Any noticeable habits or behaviors?
Example:
The student builds a bar chart showing performance across math topics. Algebra clearly trails behind geometry. A quick check of time logs shows only 2 hours spent on algebra vs. 6 hours on geometry.
Then comes a surprise: the student slept poorly before every test that focused on algebra. Hmm. That’s a connection worth noting.
Step 5: Draw Insights and Recommend Actions
The next phase of the data analytics framework involves interpreting the analysis and recommending practical, data-backed actions.
Here’s where everything comes together. You use your findings to identify real insights — and come up with a plan.
Instead of presenting a bunch of stats, tell a story. Help others (or yourself) see what needs to change.
Example:
The student identifies two core issues:
1. Not enough time spent on algebra.
2. Poor sleep before key tests.
Their new plan? Practice algebra daily for an hour. And prioritize 8 hours of sleep before tests. A solid, data-backed strategy.
Step 6: Implement, Monitor, and Iterate
The final step in the data analytics framework focuses on implementing the plan, tracking results, and refining the approach based on outcomes.
Great insights mean nothing if you don’t act on them. So now it’s time to execute your plan — and track what happens.
See what’s working and adjust as needed. Remember, data analytics isn’t about one big “aha” moment. It’s a cycle.
Example:
The student follows their new study and sleep routine. A few weeks later, the next test shows a 20% jump in algebra scores. But they still struggle with word problems. So, the plan evolves again: more practice on problem-solving.
That’s real progress — one insight, one tweak at a time.
Final Words
Let’s face it — diving into data can feel like wandering in a jungle. But with the right framework, you’ve got a map. You know where to start, what to look for, and how to turn numbers into next steps.
This six-step approach makes analytics less intimidating and a whole lot more approachable:
1. Define the problem
2. Gather relevant data
3. Clean and prepare the data
4. Explore and analyze
5. Draw insights and recommend actions
6. Implement and monitor
At Enqurious, we simplify the process of turning raw data into actionable insights. Our platform empowers you to tackle real-world problems through structured, role-based learning. Just like the data analytics framework we’ve outlined, we guide you step-by-step, providing the tools and knowledge to not only analyze data but to transform it into meaningful decisions that drive impact. With Enqurious, learning becomes an engaging, hands-on journey, where you develop the skills to excel in today’s data-driven world.
Ready to dive into the world of data analytics? Start learning with Enqurious today!